
Retail Product Placement using AI-ML
Increased sales of watermelons and shoe-care products by changing aisle placement.
As a result, watermelon sales increased by 18%, and shoe-care product sales were up by 22%. This increase was a direct result of changing the aisle placement of both products.
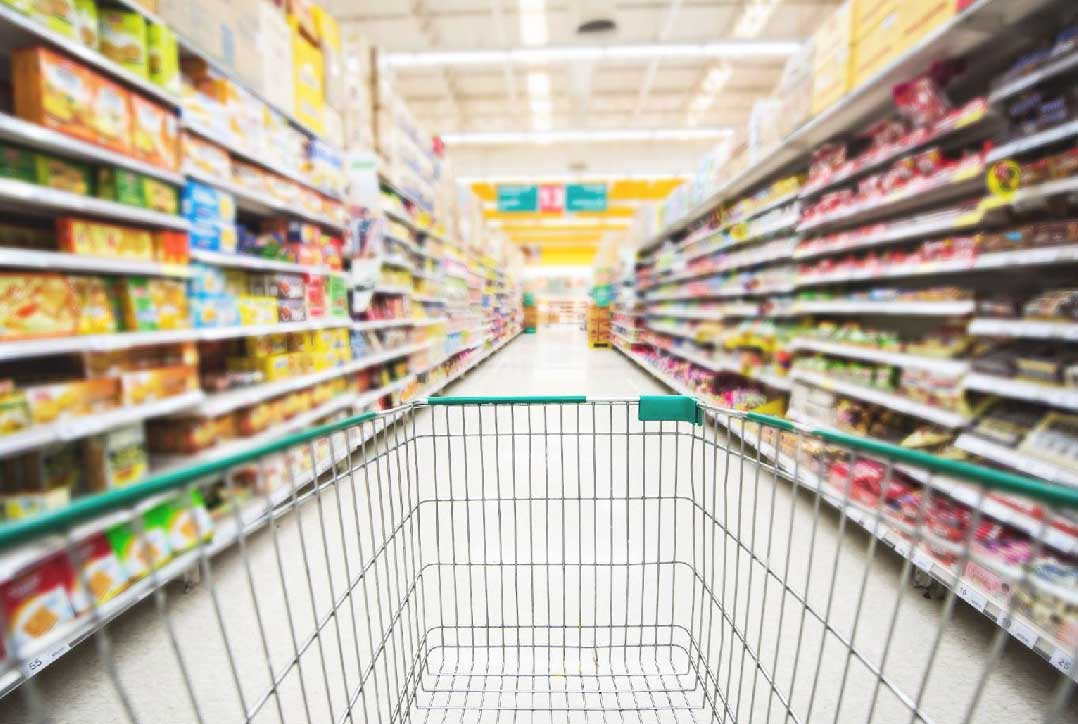
SITUATION
One of the largest retailers wanted to implement various low-cost ROI actions to increase revenue. They had data for consumer behavior and buying patterns from over 4000 company-owned stores.
CONTRIBUTING FACTORS
- The solution was a result of AI-driven data analysis and various models of existing consumers.
- These models were effective, as determining factors that were not understood by humans, were successfully identified by data variance AI.
CHALLENGES
- Database AIs and Aisle modeling systems in the market were not cost-effective.
- Their NLP models could not provide effective and actionable solutions.
SOLUTION
We custom-built a virtual data lake at a very low cost and performed AI-driven data variance analysis. Based on aisle placement in 4000+ store, completely new dimensions of consumer behavior was discovered.
EXAMPLE
Ergonomic complexity of end-usage of a product, fragments the demographic in strong buying patterns. These micro demographics were very specific in time. A small Aisle change or product visibility modifications could significantly influence buying patterns.
